numpy.random
is a sub-package of NumPy
for working with random numbers and is somewhat similar to Python’s standard library random
but instead works with numpy arrays. It can create arrays of random numbers from various statisitical probability distributions and also randomly sample from arrays or lists. The numpy.random
module is frequently used to fake or simulate data which is an important tool in data analysis, scientific research, machine learning and other areas. The simulated data can be analysed and used to test methods before applying to the real data.
A NumPy ndarray
can be created using the NumPy array
function which takes any sequence-like objects such as lists, nested lists etc. Other NumPy functions can create new arrays including zeros
, ones
, empty
, arange
, full
and eye
among others as detailed in the numpy quickstart tutorial. NumPy’s random module also creates ndarray objects.
Some key points about any of NumPy’s ndarray
objects which are relevant to the numpy.random
module:
- The data in an
ndarray
must be homogeneous, that is all of it’s data elements must of the same type. - Arrays have a
ndim
attribute for the number of axes or dimensions of the array - Arrays have a
shape
attribute which is a tuple that indicates the size of the array in each dimension. The length of theshape
tupple is the number of axes that the array has. - Arrays have a
dtype
attribute which is an object that describes the data type of the array. - The
size
attribute is the total number of elements in the array.
Python’s standard library random
already provides a number of tools for working with random numbers. However it only samples one value at a time while numpy.random
can efficiently generate arrays of sample values from various probability distributions and also provides many more probability distributions to use. The numpy.random
module is generally much much faster and more efficient than the stdlib random
module particularly when working with lots of samples, however the random
module may be sufficient and more efficient for other simpler purposes.
As well as being able to generate random sequnces, numpy.random
also has functions for randomly sampling elements from an array or sequence of elements, numbers or otherwise.
Both of these random
modules generate pseudorandom numbers rather than actual random numbers. Computer programs are deterministic because their operation is predictable and repeatable. They produce outputs based on inputs and according to a set of predetermined steps or rules so therefore it is not really possible for a computer to generate truly random numbers. These random modules implements pseudorandom numbers for various distributions that may appear random they are not truly so.
According to wolfram mathworld a random number is a
A random number chosen as if by chance from some specified distribution such that selection of a large set of these numbers reproduces the underlying distribution. Almost always, such numbers are also required to be independent, so that there are no correlations between successive numbers. Computer-generated random numbers are sometimes called pseudorandom numbers, while the term "random" is reserved for the output of unpredictable physical processes. When used without qualification, the word "random" usually means "random with a uniform distribution." Other distributions are of course possible.
Being able to randomly generate or select elements from a set has many uses in software applications such as gaming. Randomness has many uses in science, art, statistics, cryptography, gaming, gambling, and other fields. For example, random assignment in randomized controlled trials helps scientists to test hypotheses, and random numbers or pseudorandom numbers help video games such as video poker. Wikipedia: Applications of randomness
The ability to generate sets of numbers with properties from a particular probability distribution is also very useful for simulating a dataset, maybe prior to the real dataset becoming available but also for demonstrating of and learning statistical and data analysis concepts.
Data Analytics and Machine learning projects frequently use random sampling on actual datasets for testing and evaluation analytical methods and algorithms. A set of samples of data are studied and then use to try and predict the properties of unknown data. In machine learning, a dataset can be split into training and test set where the dataset is shuffled and a classifier is built with a randomly selected subset of the dataset and the classifier is then tested on the remaining subset of the data. A train-test split is used for model selection and cross validation purposes.
According to scikit-learn tutorial: Machine learning is about learning some properties of a data set and then testing those properties against another data set. A common practice in machine learning is to evaluate an algorithm by splitting a data set into two. We call one of those sets the training set, on which we learn some properties; we call the other set the testing set, on which we test the learned properties.
The machine learning algorithms in the scikit-learn
package use numpy.random
in the background. There is a random element to the train_test split as it uses numpy.random
to randomly choose elements for the training array and the test array. Cross validation methods such as k-fold validation randomly sploit the original dataset into training and testing subset many times.
Read the original section of the project notebook: Task 1
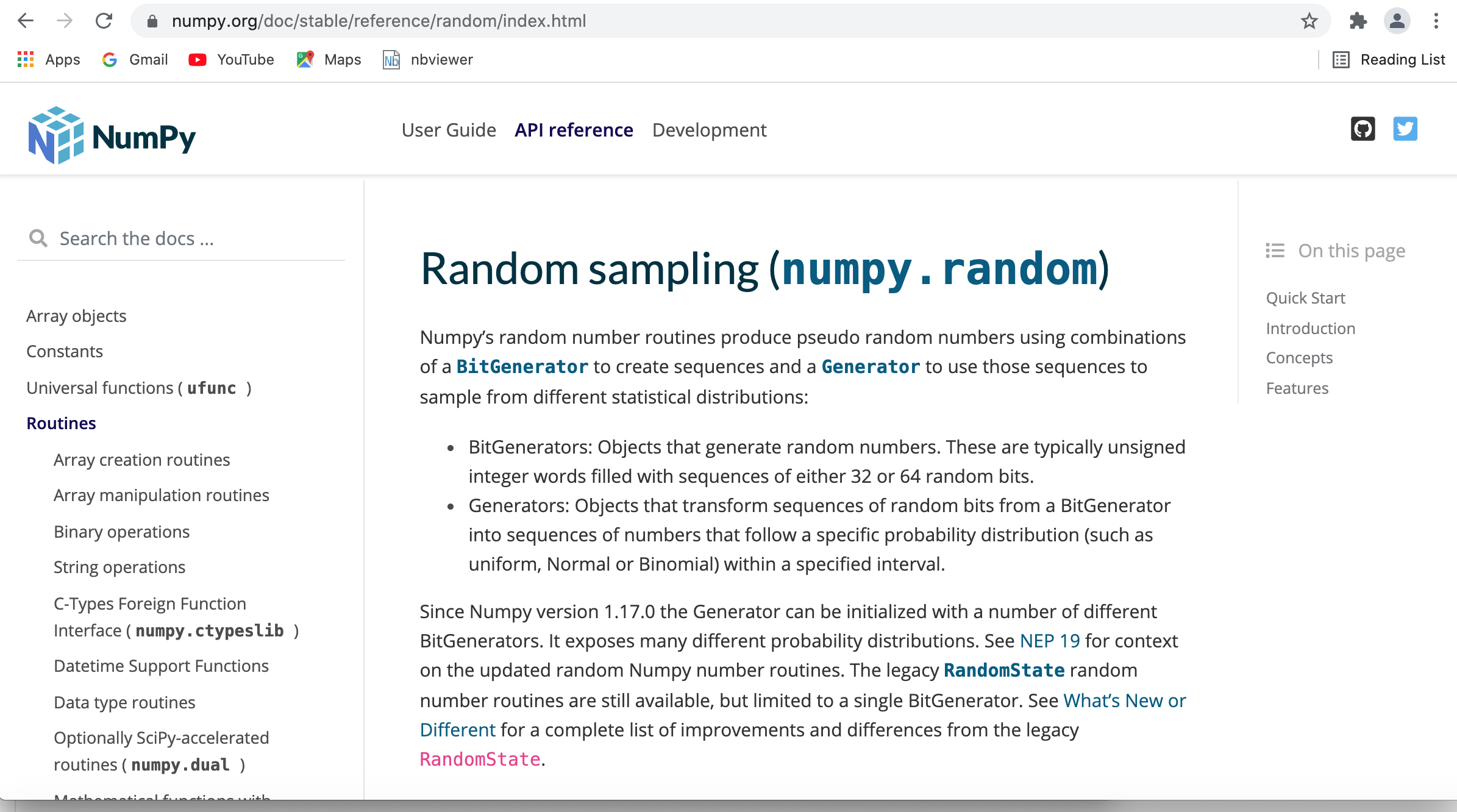